Faced with Serious Challenges, Agriculture Looks to Autonomous Technology for Timely Real-World Answers
The financial forecast for U.S. farmers is promising, with the U.S. Department of Agriculture projecting 2022 net farm income to rise by 14 percent over 2021—nearly $20 billion. While this represents the highest net farm income since 1973 (adjusted for inflation), and a record high for net cash farm income ($11 billion), the big picture looks a bit different. Farmers continue to face serious challenges on a global scale, including a daunting shortage of workers, increased competition, and the pressures of feeding a growing global population and adjusting to climate change.
Autonomous technology offers real-world solutions to these challenges today, including one vital advantage: automated agricultural equipment can work 24/7, increasing profits as well as safety, even with smaller staffs. The venerable John Deere Company introduced a fully autonomous tractor in 2022, bringing computer vision to agricultural equipment for the first time. The company has also developed autonomous sprayers as a more targeted and cost-effective method for distributing pesticides.
This is just the beginning. Along with the benefits of precision fertilization, automated agricultural equipment offers many major opportunities for dealing with a shifting climate and rough terrain. The move toward full autonomy is speeding up and gaining ground.
Self-driving equipment in the field
Harvesting crops is nothing like driving around town or along a highway, and autonomous farm equipment demands unique solutions. Just as it does with automotive autonomy, 3D sensing lies at the core of farming autonomy—it’s what enables a machine to build an accurate picture of its surroundings. A self-driving tractor or harvester must navigate from a parking area to the field, make its way up and down the field row by row while turning at the end of each row to enter the next one, and then return safely to the parking area, all without colliding with anything or anyone. There are no lane markers in the field, so the equipment operates without the visual guidance an automotive system would have.
Harvesting offers many opportunities for automation. One example is positioning: both positioning the vehicle in the field and within a row of crops, and positioning a component in relation to another component or vehicle. For example, as a grain harvester cuts and processes wheat, grain pours out of a chute into a following vehicle that collects the grain in a giant bin as it trails alongside the harvester. The alignment of the spout is critical as both vehicles move independently over bumpy fields that cause extreme vibration. It’s also important for automated tractors to differentiate between crops and weeds as they move through the fields.
In addition to working in high vibration environments, other criteria unique to autonomous farming include nighttime operation, and operation in dusty environments. These low-visibility conditions both have an impact on the choice of 3D sensor system, and are critical to the calculus of autonomous farming. Nighttime operation is important as this opens up the idea of 24-hour farming, which increases yield and efficiency. Also, farms are inherently dusty, and many optical sensor systems, like lidar and cameras, have reduced performance in degraded visual environments.
Autonomous technology at work
A variety of 3D sensor types can be used on autonomous vehicles. Single and multi-camera systems are considered “passive” optical sensors, while lidar and radar are considered active sensors because they emit energy that bounces off objects. Each of these systems handle vibration, dust, and low light differently, and each has advantages and disadvantages, including significant cost differences. Some have much longer lifetimes than others as well.
Stereo vision is one sensing technique that is well-suited for autonomous farming. It uses two or more cameras to estimate the distance between the vehicle and objects surrounding the vehicle. New advanced stereo vision systems therefore enable the cameras to be mounted independently on the vehicle and offer precise and long-range depth sensing. (Many trucks already have cameras installed for safety and driver assistance features, which makes it easier to integrate stereo vision systems into existing hardware). This approach uses software to continuously calibrate the cameras with respect to one another, ensuring exact alignment at all times, making these systems robust to vibration. Due to its many moving parts, lidar operates less effectively in rugged environments, and at higher cost.
Because stereo vision compares high-resolution images taken from multiple cameras, these systems work well in changing lighting conditions, and tend to be significantly more resilient to dust and bad weather as compared with active systems like lidar. Lidar, which sends out light photons and measures their return time, is susceptible to particulates in the air that scatter the laser emissions. Significantly, the vision-based approach is better at identifying and monitoring weeds in real time as the tractor works.
Another important benefit of stereo vision sensors is their use of standard, off-the-shelf cameras, which today ship in the billions of units and are extremely high resolution, photo-sensitive, and low-cost. These benefits, along with the 15-year average lifetime of cameras today, make camera-based 3D sensors a good fit for the autonomous farming market.
The future of farming
The benefits of advanced stereo vision—cost, performance, robustness, range—position the technology as well-suited to the 3D sensing needs of autonomous agricultural equipment. Whether automating the navigation and operation of a harvester, or using the technology to position pesticide sprayers, differentiate between weeds and crops, and calibrate cameras, there is no doubt that advanced stereo vision will be fundamental to the evolution of industrial automated farming.
Comments (0)
This post does not have any comments. Be the first to leave a comment below.
Featured Product
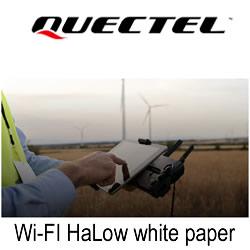