Machine-Vision Technology Shows Promise to Reduce Herbicide Use
Farmers and land-managers seeking to reduce their herbicide applications now have another promising option via machine-vision technology. That's the summary from a recently published research article in Weed Technology, a journal of the Weed Science Society of America (WSSA).
"Based on these results with specific machine settings, targeted applications programs could reduce the herbicides applied while providing comparable weed control to traditional broadcast applications," says Tristen Avent, University of Arkansas, Senior Graduate Assistant in the Crop, Soil, and Environmental Sciences Department, and corresponding author of the study. "More specifically, our research showed that, on average, this technology's targeted sprays saved a range of 28.4 to 62.4% on postemergence herbicides compared to traditional broadcast applications."
According to the Foreign Agriculture Service, total production costs for row crop farms increased in the United States by 26.6% from 2017 to 2022, and chemicals averaged 7.7% of the total cost (2023). "With continually increasing crop production costs, it's vital for producers to find technologies that can both reduce their costs and improve profitability," says Avent. "In addition to significant opportunities to lower herbicide costs and improve profits, our research also showed that the targeted applications from machine-vision technology can be utilized to provide some soybean health benefits and improve environmental stewardship."
In this study, researchers used John Deere's See & Spray™ Technology to compare machine-vision targeted spray technology with traditional broadcast applications. Researchers conducted the experiments for two years in Keiser, AR, and Greenville, MS, to compare residual herbicide timings and targeted spray applications versus traditional broadcast herbicide programs in glyphosate/glufosinate/dicamba-resistant soybean.
"Results may vary with other machine-vision technologies or with different herbicide programs and machine weed-detection sensitivity settings," notes Avent. "Future research will consider these factors, among others."
More information about machine-vision opportunities to reduce herbicide use can be found in the article, "Comparing Herbicide Application Methods with See & Spray™ Technology in Soybean." The research is featured in the Volume 38, 2024, issue of Weed Technology, a Weed Science Society of America journal, published online by Cambridge University Press.
About Weed Technology
Weed Technology is a journal of the Weed Science Society of America, a nonprofit scientific society focused on weeds and their environmental impact. Weed Technology publishes original research and scholarship in the form of peer-reviewed articles focused on understanding how weeds are managed. The journal focuses on applied aspects concerning weed management in agricultural systems, weed/crop management systems, new weed problems, new technologies for weed management, herbicides used to manage undesired vegetation, and special articles emphasizing technology transfer to improve weed control. To learn more, visit http://www.wssa.net.
Comments (0)
This post does not have any comments. Be the first to leave a comment below.
Featured Product
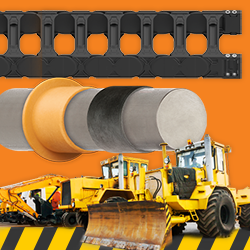